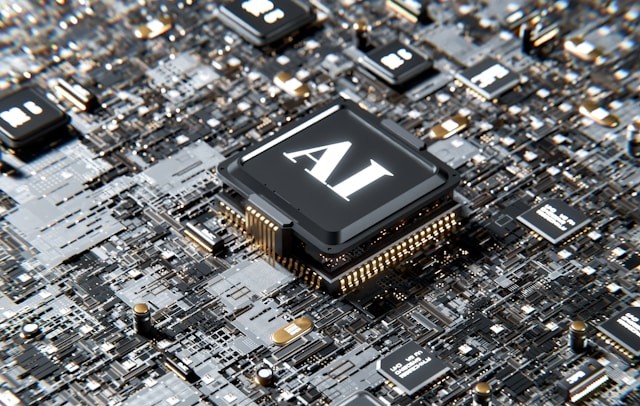
Introduction
The artificial intelligence landscape is undergoing a profound transformation. While cloud-based AI has dominated headlines for years, a quieter revolution has been taking place at the periphery of our networks. Edge AI—the deployment of artificial intelligence algorithms directly on end devices rather than in centralized cloud environments—is rapidly emerging as one of the most significant technological shifts in the Internet of Things (IoT) ecosystem.
This approach to AI implementation brings computation closer to where data is generated, enabling faster processing times, enhanced privacy, and reduced bandwidth requirements. As we progress through 2025, Edge AI is moving from experimental deployments to mainstream adoption across multiple industries, reshaping how we think about intelligent systems.
What is Edge AI?
Edge AI refers to the implementation of artificial intelligence algorithms directly on edge devices—smartphones, sensors, cameras, wearables, and other IoT endpoints—rather than relying on cloud-based processing. These systems can perform data analysis, make decisions, and take actions locally, without constant connectivity to centralized servers.
The fundamental advantage of Edge AI lies in its ability to process data where it’s created. This architectural shift eliminates the need to transmit vast amounts of raw data to the cloud, addressing latency, bandwidth, privacy, and reliability concerns that have limited traditional cloud AI implementations.
Key Technological Enablers
Several technological advances have converged to make Edge AI commercially viable:
- Neural Processing Units (NPUs): Specialized hardware designed specifically for AI workloads has dramatically improved energy efficiency. The latest generation of NPUs can handle complex neural network operations while consuming minimal power, making them ideal for battery-powered devices.
- Model Compression Techniques: Innovations in quantization, pruning, and knowledge distillation have reduced the size of AI models without significant performance degradation. Models that once required powerful cloud servers can now run efficiently on resource-constrained devices.
- Federated Learning: This approach allows edge devices to collaboratively learn a shared model while keeping all training data local. Devices only share model updates rather than raw data, preserving privacy while still benefiting from collective intelligence.
- TinyML Frameworks: Development tools like TensorFlow Lite, ONNX Runtime, and the newer EdgeML+ have simplified the process of deploying AI models to edge devices, making the technology accessible to a broader range of developers.
Real-World Applications
Edge AI is driving innovation across numerous sectors:
Smart Manufacturing
Manufacturing facilities are deploying Edge AI-powered visual inspection systems that can detect defects in real-time without sending sensitive production data to external servers. These systems analyze thousands of products per minute with millisecond response times, something cloud-dependent systems cannot match due to latency constraints.
The latest implementations incorporate adaptive learning, allowing inspection parameters to adjust automatically as production conditions change, reducing false positives and continuously improving accuracy without human intervention.
Healthcare Monitoring
Wearable health devices now incorporate sophisticated Edge AI capabilities for continuous health monitoring. The newest generation of smart watches and medical wearables can detect irregular heartbeats, monitor blood oxygen levels, and even identify early signs of conditions like sleep apnea or atrial fibrillation—all processed locally on the device.
This local processing approach ensures that sensitive health data remains private while still enabling potentially life-saving alerts when abnormalities are detected.
Smart Cities and Infrastructure
Cities are implementing Edge AI-powered traffic management systems that can adapt to changing conditions in real-time. Intelligent cameras at intersections analyze traffic flow patterns locally, dynamically adjusting signal timing to reduce congestion and improve safety.
Similar applications are emerging in infrastructure monitoring, where Edge AI enables continuous structural health assessment of bridges, dams, and buildings without generating massive data streams that would overwhelm network connections.
Autonomous Vehicles
Perhaps the most demanding Edge AI application is in autonomous vehicles, where decision-making latency can be a matter of life and death. The latest autonomous systems use distributed Edge AI architectures where multiple specialized neural networks handle different aspects of driving—object detection, path planning, and driver monitoring—all operating simultaneously within the vehicle.
This approach enables vehicles to function safely even when network connectivity is limited or unavailable, a critical requirement for widespread deployment.
Business Impact and Market Growth
The business case for Edge AI continues to strengthen as implementations demonstrate clear ROI across multiple dimensions:
- Reduced operational costs: By processing data locally, organizations can reduce cloud computing expenses and bandwidth costs by up to 70% for AI-intensive applications.
- Enhanced customer experiences: Lower latency enables more responsive applications and services, particularly important for consumer-facing IoT devices.
- New business models: Edge AI enables entirely new categories of products and services that weren’t previously possible due to privacy, connectivity, or latency constraints.
Industry analysts project the Edge AI market to grow from $11.3 billion in 2024 to approximately $38 billion by 2029, reflecting a compound annual growth rate of 27.5%. This growth is being driven not just by technology giants but also by specialized startups focusing on industry-specific Edge AI solutions.
Challenges and Limitations
Despite its promise, Edge AI faces several significant challenges:
- Resource Constraints: Edge devices still have limited computational capabilities compared to cloud environments, restricting the complexity of models that can be deployed.
- Model Maintenance: Updating AI models across thousands or millions of distributed edge devices presents logistical challenges for version control and synchronization.
- Security Concerns: Edge devices may be physically accessible to malicious actors, creating new attack vectors for adversarial examples or model extraction attacks.
- Development Complexity: Creating efficient Edge AI solutions requires specialized knowledge spanning hardware optimization, model compression, and embedded systems development.
The Future of Edge AI
Looking ahead, several trends will shape the evolution of Edge AI:
Hybrid Edge-Cloud Architectures
Rather than choosing between edge or cloud processing, the most effective implementations will leverage both approaches. Routine processing will happen at the edge, while complex or novel scenarios might trigger cloud-based analysis. This hybrid approach combines the latency benefits of edge processing with the computational power of the cloud.
Distributed Intelligence Networks
Future Edge AI systems will increasingly operate as collaborative networks rather than isolated endpoints. Devices will share insights and model improvements while maintaining data privacy, creating collectively intelligent systems that are greater than the sum of their parts.
Energy-Harvesting Edge AI
As energy efficiency improves, we’re beginning to see the emergence of self-powered Edge AI devices that can harvest energy from their environment—solar, thermal, kinetic, or RF energy—eliminating battery constraints entirely for certain applications.
Edge AI as a Service
Cloud providers are developing specialized offerings that simplify the development, deployment, and management of Edge AI solutions. These platforms provide the tools and infrastructure to bridge the gap between cloud and edge environments, making Edge AI more accessible to organizations without specialized expertise.
Conclusion
Edge AI represents a fundamental shift in how we architect intelligent systems, moving processing closer to data sources to overcome the limitations of cloud-centric approaches. As hardware capabilities improve, development tools mature, and implementation patterns emerge, Edge AI will become an increasingly central component of the IoT landscape.
For organizations developing IoT strategies, incorporating Edge AI capabilities should be a priority consideration. Those who successfully leverage this technology will gain significant advantages in operational efficiency, customer experience, and the ability to deliver innovative products and services.
The intelligence of the future won’t just reside in distant data centers—it will be embedded in the devices all around us, working silently and efficiently at the edge of the network.